DataLab

Quinten group’s R&D laboratory
To this end, we have a dedicated structure called the DataLab, which is largely focused on R&D in artificial intelligence to produce new tools, methodologies, and results aimed at integrating the latest and most efficient approaches.
The team, composed of varied profiles (PhDs, postdocs, researchers, engineers, and consultants), has a triple objective:
- To propose innovative solutions in the field of AI for healthcare and finance;
- To ensure the technical quality of our deliverables on client projects;
- To conduct high-quality scientific monitoring and disseminate it internally.
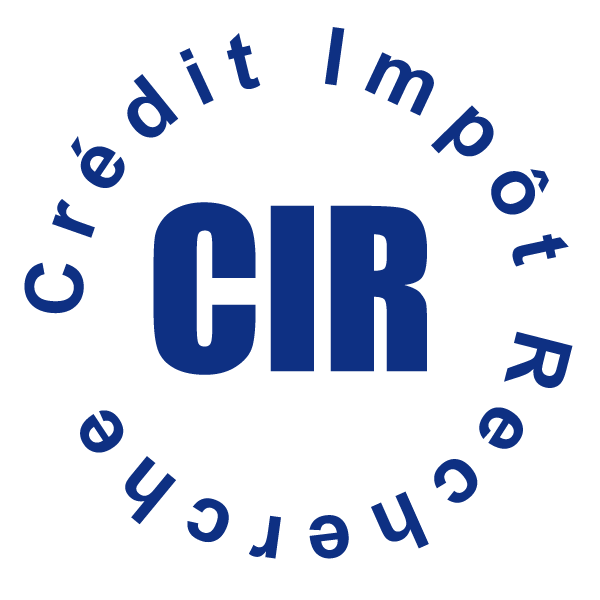
MULTI-DISCIPLINARY APPROACH TO ARTIFICIAL INTELLIGENCE
Q-Finder Quinten’s expertise in artificial intelligence is based on five specialized areas, which form the core of our R&D. This focus is the result of the company’s historical experience as a pioneer in AI in healthcare, which has allowed us to accumulate significant experience in this particular field, as well as our openness to other methodologies enabled by our multi-sectoral approach.
Real-world data
The first area focuses on the exploitation of real-world data, which requires a high level of expertise due to its complexity and technicality.
The development of complementary approaches based on biostatistics (Bayesian models, etc.) and deep learning (Recurrent Neural Networks, AutoEncoders) allows us to handle a wide range of use cases with state-of-the-art performance, such as predictive diagnosis, etc.
To this end, we rely on various internally developed algorithms such as Q-Finder, and the Medical Code Generator.
Natural Language Processing (NLP)
The second area is devoted to Natural Language Processing (NLP), with a specialization in biomedical documents (physician reports, biomedical literature, patient comments, etc.), particularly in French.
The development of deep learning algorithms (transformers) specifically trained on specialized corpora such as AliBERT allows us to offer extremely performant solutions for various tasks, such as detection of side effects, extraction of biomedical entities in oncology, classification of medical reports, etc.
Sequence processing
Client and patient journeys are naturally represented as a sequence of events (hospital or doctor visits for a patient, for example). Quinten has developed particular expertise around the modeling of this type of data through sequential representation, leading to the creation of proprietary algorithms such as Seqminer.
This approach allows us to obtain better performance and a finer understanding of use cases as varied as diagnosis prediction, client scoring, patient survival, clustering of disease progression, etc.
Model explainability
Most use cases handled within Quinten require an understanding of the modeled phenomena. It is therefore essential to be able to explain and interpret the predictions of our models.
In this context, we have developed several approaches specific to use cases in the healthcare and banking/insurance domains, allowing us to provide explanation elements for models at different levels of detail.
Graphs
Representing data as graphs offers particular advantages that are difficult and laborious to obtain via tabular data. Moreover, the biomedical domain is full of ontologies and classifications naturally taking the form of graphs, allowing knowledge to be structured.
At Quinten, we are working on the development of methodologies to boost the performance of our algorithms based on knowledge graphs already available or that we enrich to better respond to the use cases we handle.
OUR R&D IN FIGURES/h2>
scientific publications
%
of revenue invested in R&D
employees dedicated to research
proprietary algorithms
OUR PROPRIETARY ALGORITHM FAMILIES
Q-Finder
Q-Finder© is an algorithm for detecting subgroups within a cohort.
In the healthcare field, it identifies subgroups of patients who may respond favorably to therapy by searching for predictive factors of the disease or response to treatment.
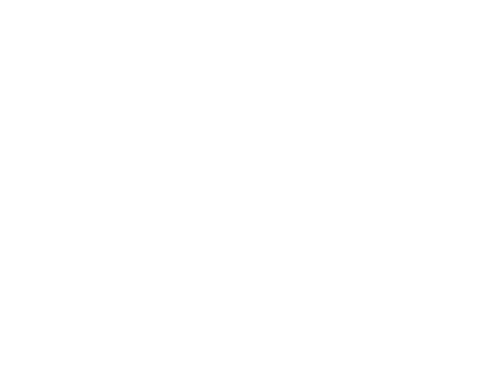
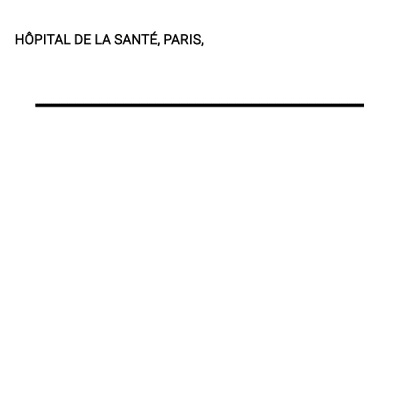
AliBERT
AliBERT is a language model specialized in the biomedical field in the French language.
Its use allows for various language analysis tasks in the medical field: detection of side effects, extraction of biomedical entities, diagnosis classification.
Medical code generator
We have developed a tool for generating medical codes (ICD10) from synonyms associated with the same class of pathology.
This tool is used to reprocess ICD10 codes found in real-life data to link them to a pathology at an aggregated level.
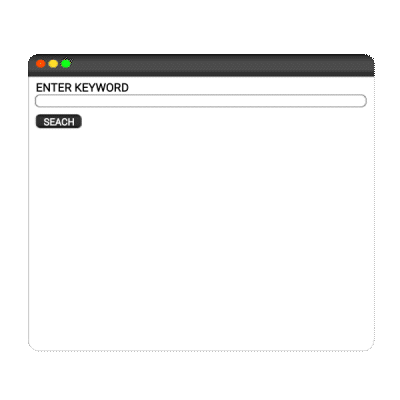
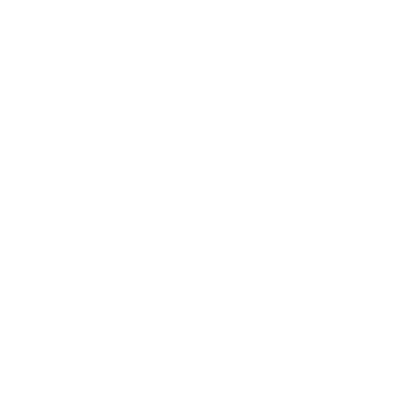
Seqminer
Seqminer is a comprehensive algorithm for detecting subsequences.
Its use is particularly relevant in the treatment of patient or customer journeys to detect common patterns identifiable in this sequential data.